Intro
Discover the 5 key differences, highlighting crucial distinctions, comparisons, and contrasts, to make informed decisions with expert analysis and insights.
The world of technology and innovation is constantly evolving, with new trends and advancements emerging every day. Two concepts that have gained significant attention in recent years are artificial intelligence and machine learning. While often used interchangeably, these terms have distinct meanings and applications. Understanding the differences between them is crucial for businesses, developers, and individuals looking to leverage these technologies to drive growth and improvement. In this article, we will delve into the 5 key differences between artificial intelligence and machine learning, exploring their definitions, applications, and implications for the future.
Artificial intelligence and machine learning are closely related, yet distinct concepts. Artificial intelligence refers to the broader field of research and development aimed at creating machines that can perform tasks that typically require human intelligence, such as reasoning, problem-solving, and learning. Machine learning, on the other hand, is a subset of artificial intelligence that focuses specifically on the development of algorithms and statistical models that enable machines to learn from data and improve their performance over time. As we explore the differences between these two concepts, it becomes clear that they have distinct applications and implications for various industries and aspects of our lives.
The importance of understanding the differences between artificial intelligence and machine learning cannot be overstated. As these technologies continue to advance and become increasingly integrated into our daily lives, it is essential to recognize their potential benefits and limitations. By grasping the fundamental distinctions between artificial intelligence and machine learning, we can better navigate the opportunities and challenges they present, ultimately unlocking their full potential to drive innovation and progress.
Definition and Scope
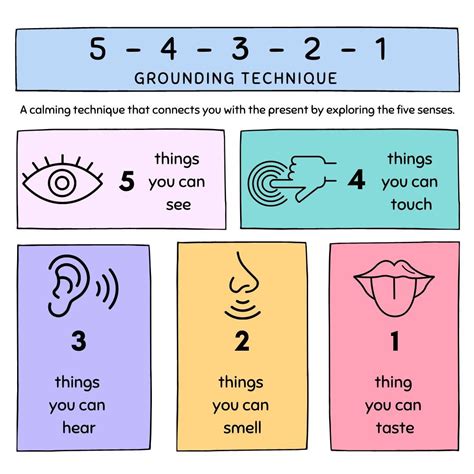
Key Characteristics of Artificial Intelligence
The key characteristics of artificial intelligence include its ability to reason, problem-solve, and learn. Artificial intelligence systems can process vast amounts of data, recognize patterns, and make decisions based on that data. They can also learn from experience, adapting to new situations and improving their performance over time. Some of the key applications of artificial intelligence include expert systems, natural language processing, and computer vision.Key Characteristics of Machine Learning
The key characteristics of machine learning include its ability to learn from data and improve its performance over time. Machine learning algorithms can be trained on large datasets, enabling them to recognize patterns and make predictions or decisions based on that data. Some of the key applications of machine learning include image recognition, speech recognition, and predictive analytics.Applications and Use Cases

Some of the key applications of artificial intelligence include:
- Expert systems: Artificial intelligence is being used to develop expert systems that can mimic the decision-making abilities of human experts in a particular domain.
- Natural language processing: Artificial intelligence is being used to develop systems that can understand and generate human language, enabling applications such as chatbots and virtual assistants.
- Computer vision: Artificial intelligence is being used to develop systems that can interpret and understand visual data from images and videos, enabling applications such as self-driving cars and facial recognition.
Some of the key applications of machine learning include:
- Image recognition: Machine learning is being used to develop systems that can recognize and classify images, enabling applications such as self-driving cars and facial recognition.
- Speech recognition: Machine learning is being used to develop systems that can recognize and transcribe spoken language, enabling applications such as virtual assistants and voice-controlled devices.
- Predictive analytics: Machine learning is being used to develop predictive models that can forecast future events and behaviors, enabling applications such as credit scoring and demand forecasting.
Real-World Examples of Artificial Intelligence
Some real-world examples of artificial intelligence include: * Virtual assistants: Virtual assistants such as Siri, Alexa, and Google Assistant use artificial intelligence to understand and respond to voice commands. * Self-driving cars: Self-driving cars use artificial intelligence to interpret and respond to visual data from cameras and sensors. * Chatbots: Chatbots use artificial intelligence to understand and respond to customer inquiries and requests.Real-World Examples of Machine Learning
Some real-world examples of machine learning include: * Image recognition: Image recognition systems use machine learning to recognize and classify images, enabling applications such as self-driving cars and facial recognition. * Speech recognition: Speech recognition systems use machine learning to recognize and transcribe spoken language, enabling applications such as virtual assistants and voice-controlled devices. * Predictive analytics: Predictive analytics systems use machine learning to forecast future events and behaviors, enabling applications such as credit scoring and demand forecasting.Techniques and Algorithms
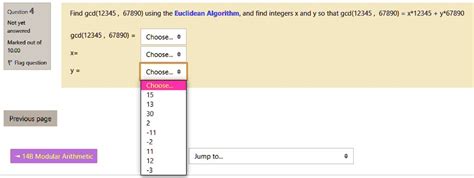
Some of the key techniques used in artificial intelligence include:
- Rule-based systems: Rule-based systems use a set of predefined rules to reason and make decisions.
- Decision trees: Decision trees use a tree-like model to classify data and make predictions.
- Neural networks: Neural networks use a complex network of interconnected nodes to recognize patterns and make decisions.
Some of the key algorithms used in machine learning include:
- Supervised learning: Supervised learning algorithms use labeled data to train models and make predictions.
- Unsupervised learning: Unsupervised learning algorithms use unlabeled data to discover patterns and relationships.
- Reinforcement learning: Reinforcement learning algorithms use rewards and penalties to train models and make decisions.
Challenges and Limitations of Artificial Intelligence
Some of the challenges and limitations of artificial intelligence include: * Data quality: Artificial intelligence systems require high-quality data to learn and make decisions. * Bias and fairness: Artificial intelligence systems can perpetuate bias and unfairness if they are trained on biased data. * Explainability: Artificial intelligence systems can be difficult to interpret and understand, making it challenging to explain their decisions and actions.Challenges and Limitations of Machine Learning
Some of the challenges and limitations of machine learning include: * Data quality: Machine learning algorithms require high-quality data to learn and make predictions. * Overfitting: Machine learning algorithms can overfit the training data, resulting in poor performance on new, unseen data. * Interpretability: Machine learning algorithms can be difficult to interpret and understand, making it challenging to explain their predictions and decisions.Future Directions and Implications
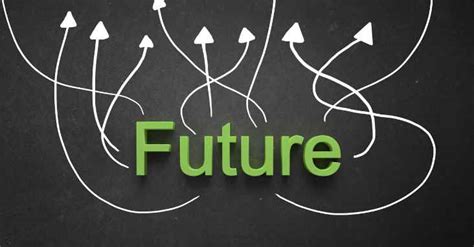
Some of the potential future directions of artificial intelligence include:
- Increased use of natural language processing and computer vision to develop more sophisticated chatbots and virtual assistants.
- Greater emphasis on developing explainable and transparent artificial intelligence systems that can provide insights into their decision-making processes.
- Increased use of artificial intelligence in healthcare and medicine to develop more personalized and effective treatments.
Some of the potential future directions of machine learning include:
- Increased use of deep learning algorithms to develop more sophisticated image and speech recognition systems.
- Greater emphasis on developing more efficient and scalable machine learning algorithms that can handle large datasets and complex problems.
- Increased use of machine learning in finance and economics to develop more accurate and reliable predictive models.
Gallery of Artificial Intelligence and Machine Learning
Artificial Intelligence and Machine Learning Image Gallery
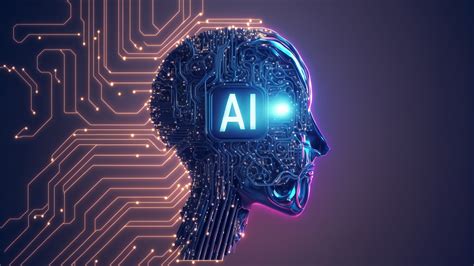
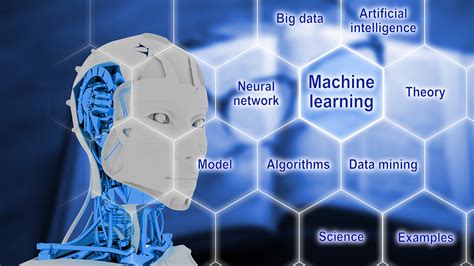
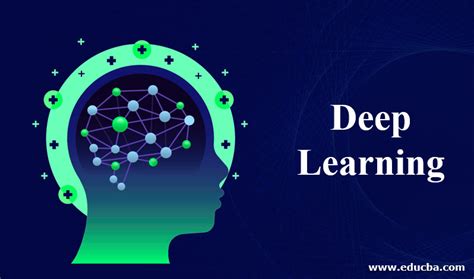
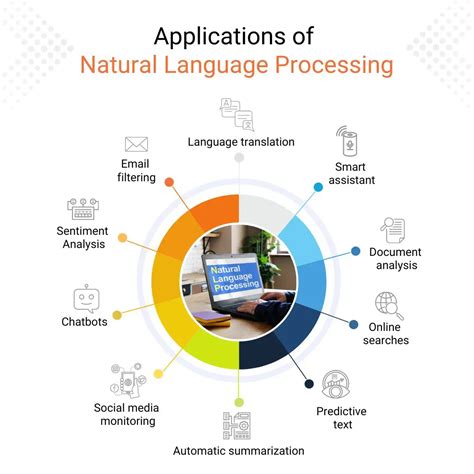
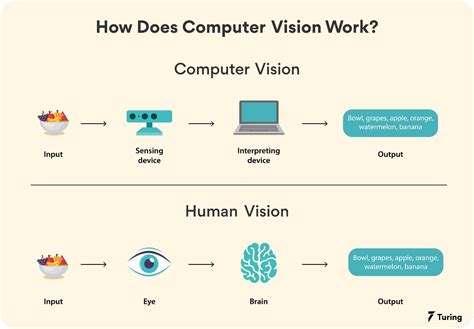
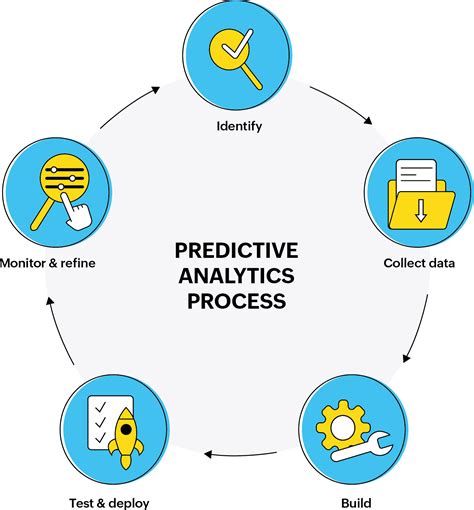
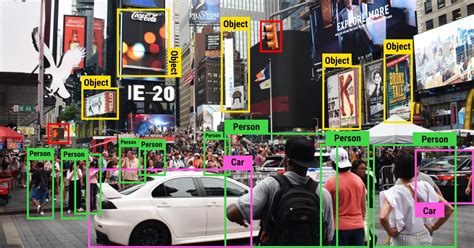
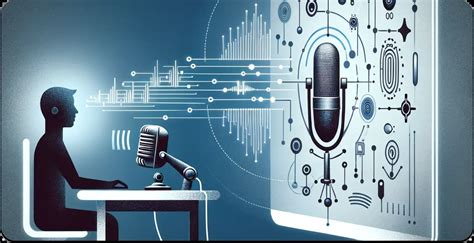
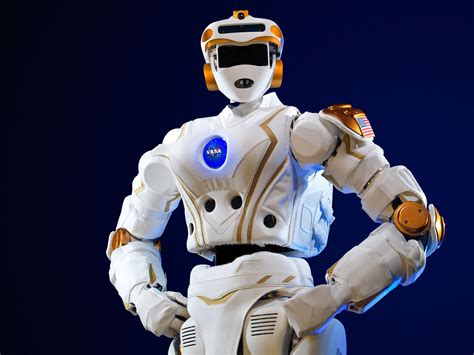
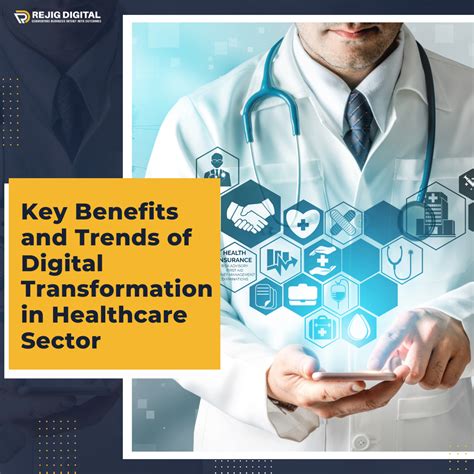
Frequently Asked Questions
What is the difference between artificial intelligence and machine learning?
+Artificial intelligence refers to the broader field of research and development aimed at creating machines that can perform tasks that typically require human intelligence, while machine learning is a subset of artificial intelligence that focuses specifically on the development of algorithms and statistical models that enable machines to learn from data and improve their performance over time.
What are some of the key applications of artificial intelligence?
+Some of the key applications of artificial intelligence include expert systems, natural language processing, and computer vision.
What are some of the key applications of machine learning?
+Some of the key applications of machine learning include image recognition, speech recognition, and predictive analytics.
What is the future of artificial intelligence and machine learning?
+The future of artificial intelligence and machine learning is significant and far-reaching, with potential applications in a wide range of industries and aspects of our lives.
How can I get started with artificial intelligence and machine learning?
+To get started with artificial intelligence and machine learning, you can explore online courses and tutorials, read books and articles, and join online communities and forums.
As we conclude our exploration of the 5 key differences between artificial intelligence and machine learning, it is clear that these technologies have the potential to drive innovation and progress in a wide range of industries and aspects of our lives. By understanding the differences between these concepts, we can better navigate the opportunities and challenges they present, ultimately unlocking their full potential to shape the future of humanity. We invite you to share your thoughts and insights on the implications of artificial intelligence and machine learning, and to join the conversation on how these technologies can be harnessed to drive positive change and improvement in our world.